LDAは、教師あり学習の1つです。カテゴリ予想に使えます。logistic regressionとLDAの使い分けはlogistic regressionは通常2カテゴリの予想に使われるのに対して、LDAは3カテゴリー以上の予想に使われます。
LDAの使い方を見てみます。使うデータは、デフォルトのirisデータです。必要なパッケージは、MASSです。
実践
library(MASS)
myiris <- iris
colnames(myiris)
[1] “Sepal.Length”
[2] “Sepal.Width”
[3] “Petal.Length”
[4] “Petal.Width”
[5] “Species”
irisにはカテゴリが3つあります。
table(myiris$Species)
setosa versicolor virginica
50 50 50
さっそく、LDAを適用させます。
lda(式, data = データ)
lda <- lda(Species~.,
data = myiris)
モデルが作れたので、モデルを使って予想したいと思います。
predict(モデル, newdata = 予測したいデータ)
pred <- predict(lda,
newdata = myiris)
predには何が入っているでしょうか。
pred$posterior
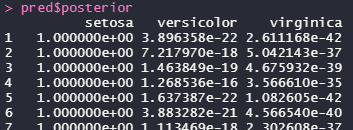
それぞれのクラスに分類される確率が入っていました。
pred$class
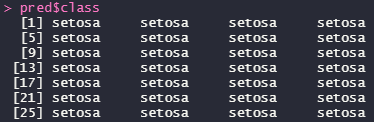
予想されるカテゴリーが入っていました。
pred$x
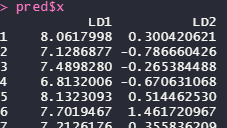
LDAの計算でつかわれる判別係数です。PCAみたいですね。
グラフを書いてみます。
library(tidyverse)
tibble(LD1 = pred$x[,1],
LD2 = pred$x[,2],
class = pred$class)%>%
ggplot()+
geom_point(aes(x=LD1,y=LD2,color=class))+
geom_density2d(aes(x=LD1,y=LD2),color="grey")+
theme_minimal()
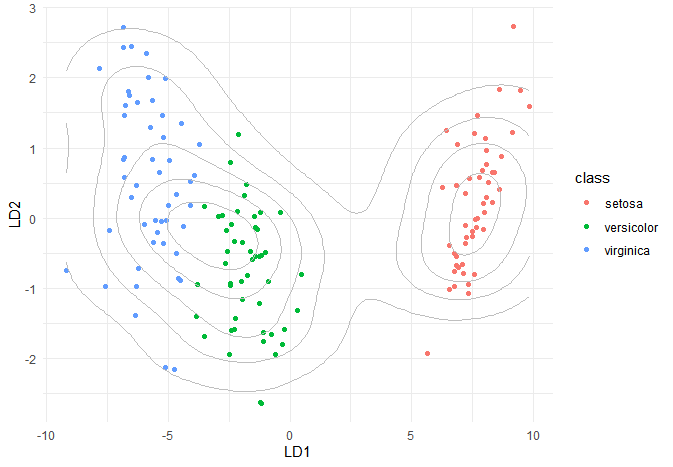
綺麗に分けられていますね。
accuracyを見てみます。
table(true=myiris$Species,
prediction = pred$class)
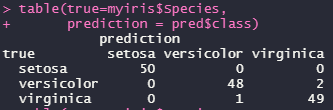
Accuracy = (50+48+49)/(50+48+1+2+49) = 0.98
なかなかいい成績ですね!